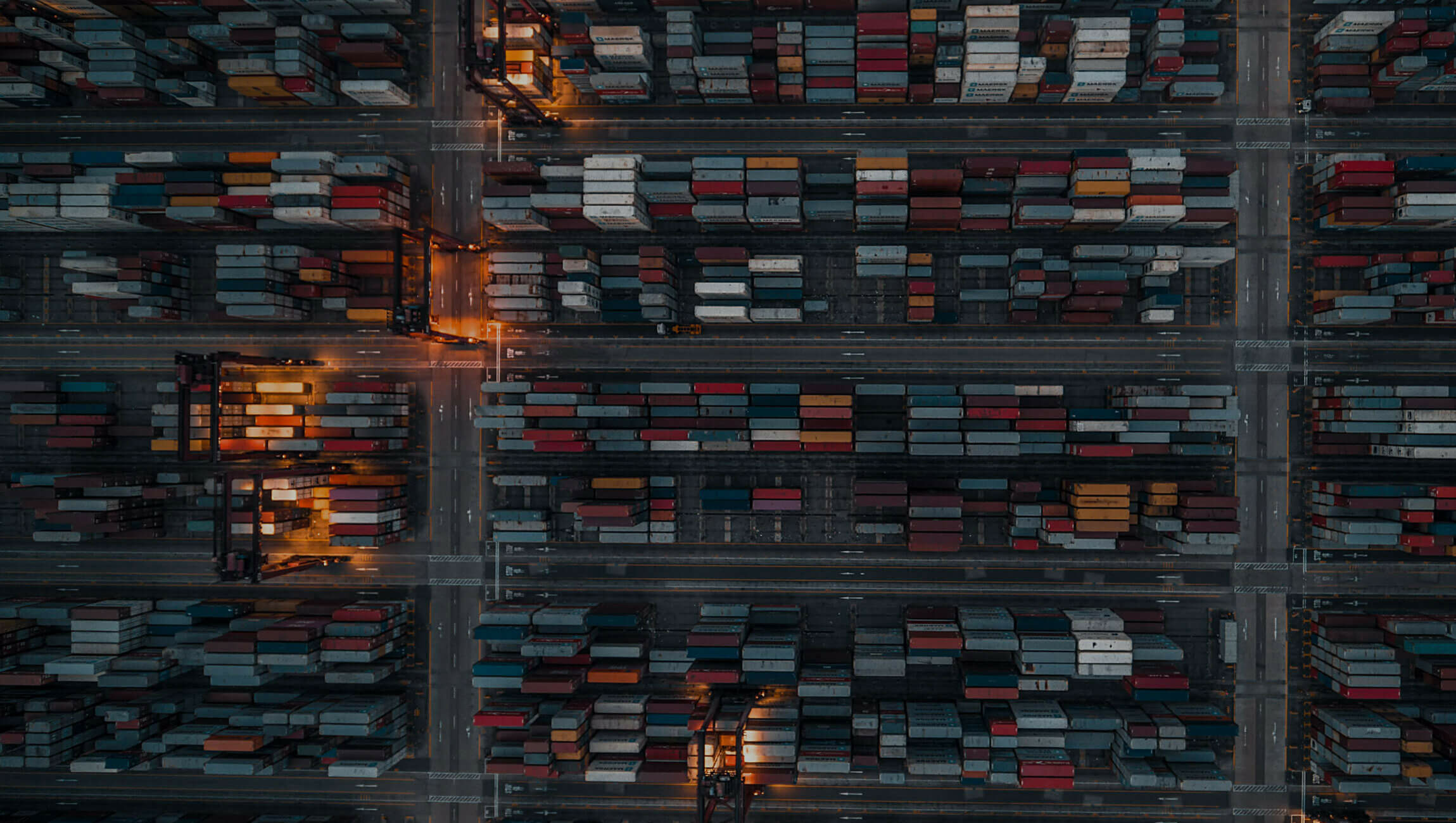
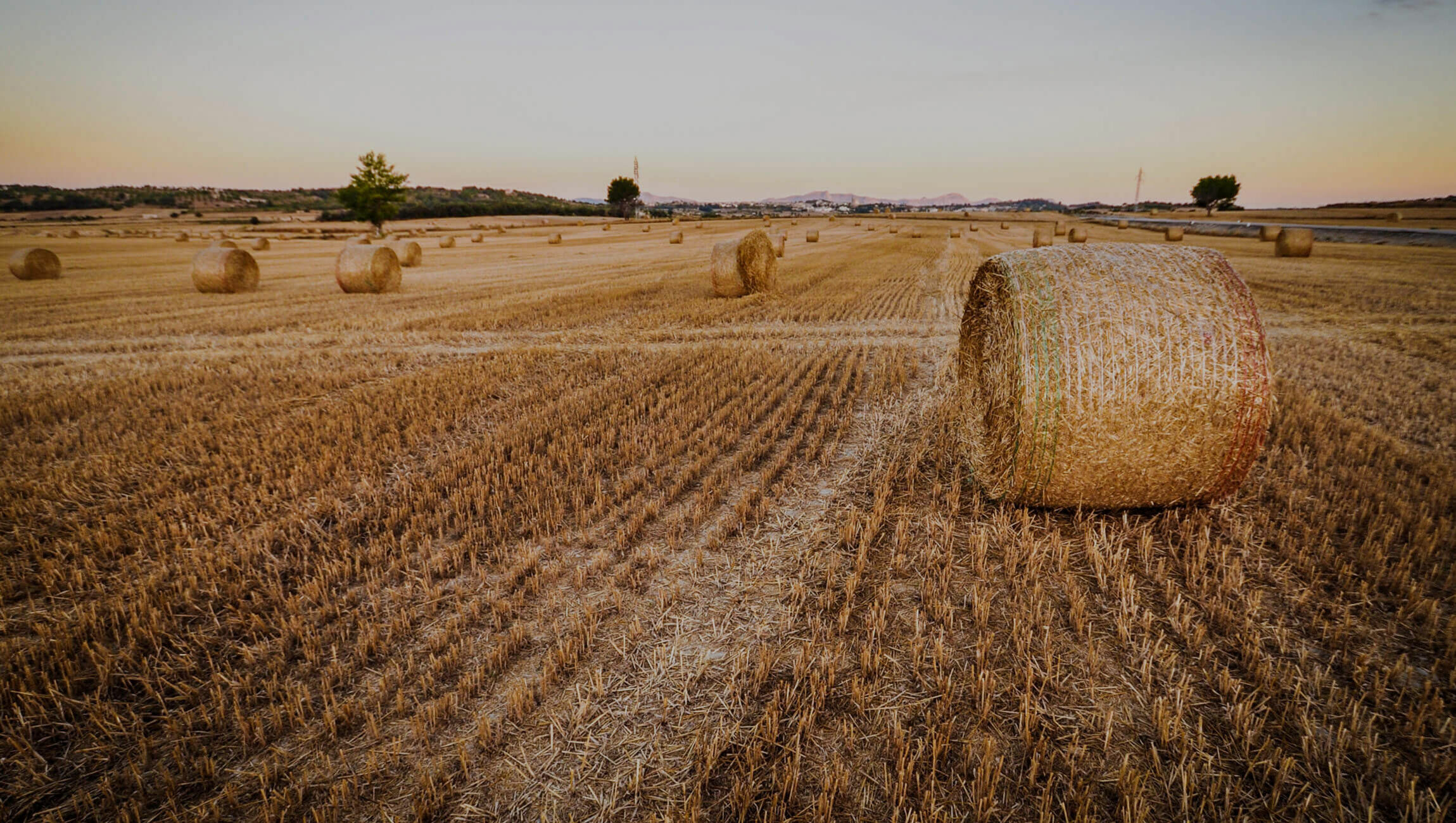
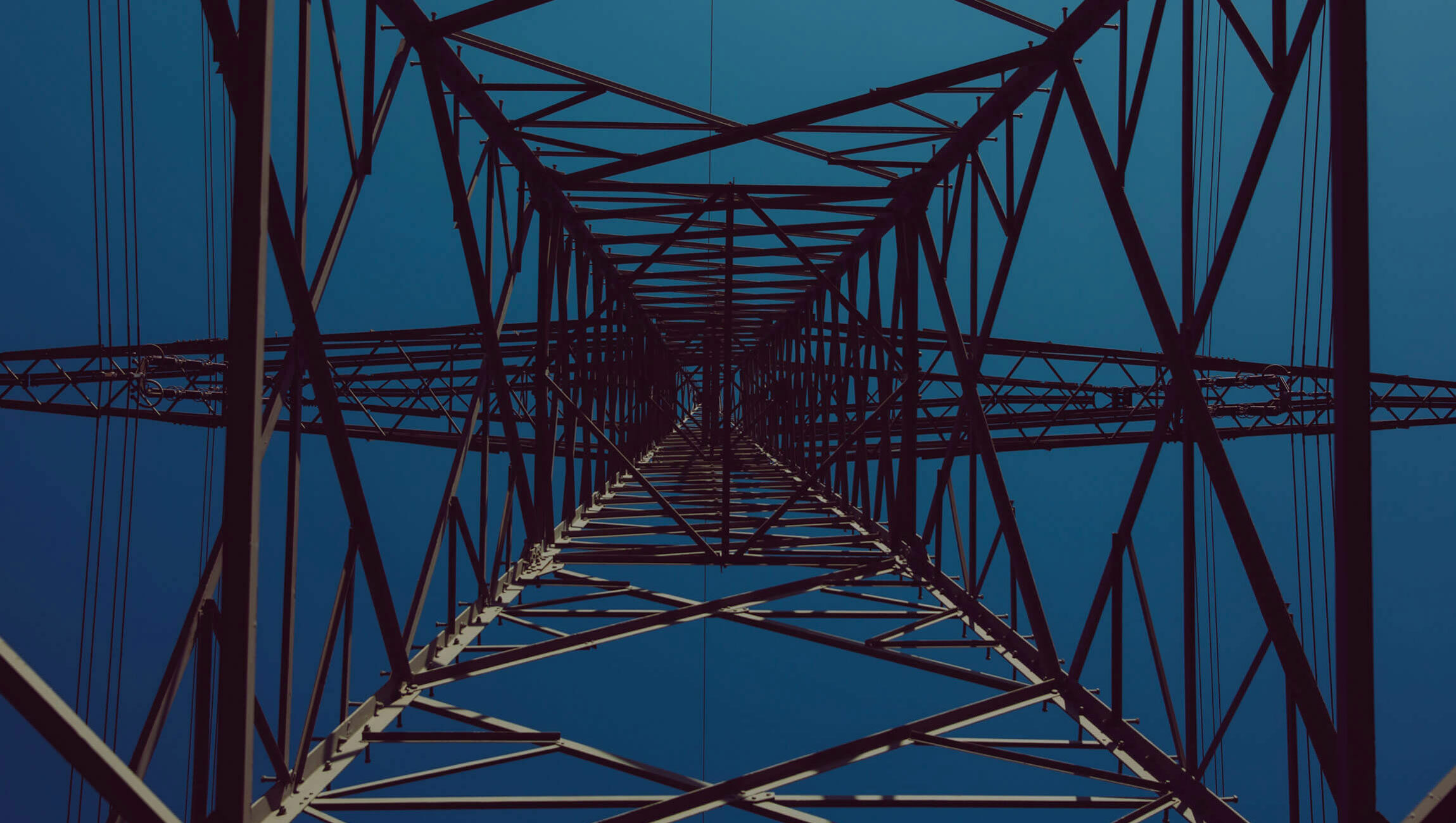
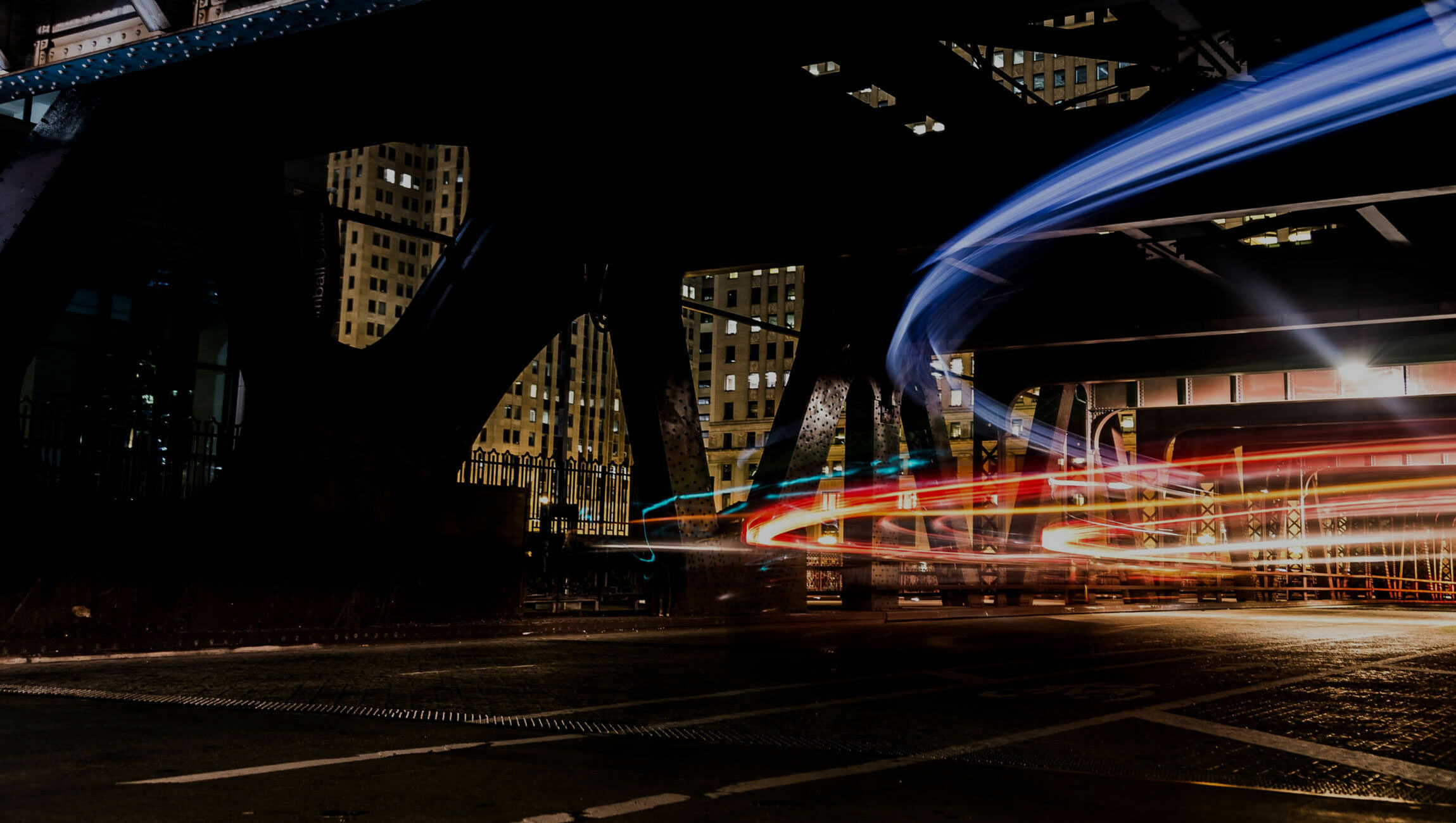
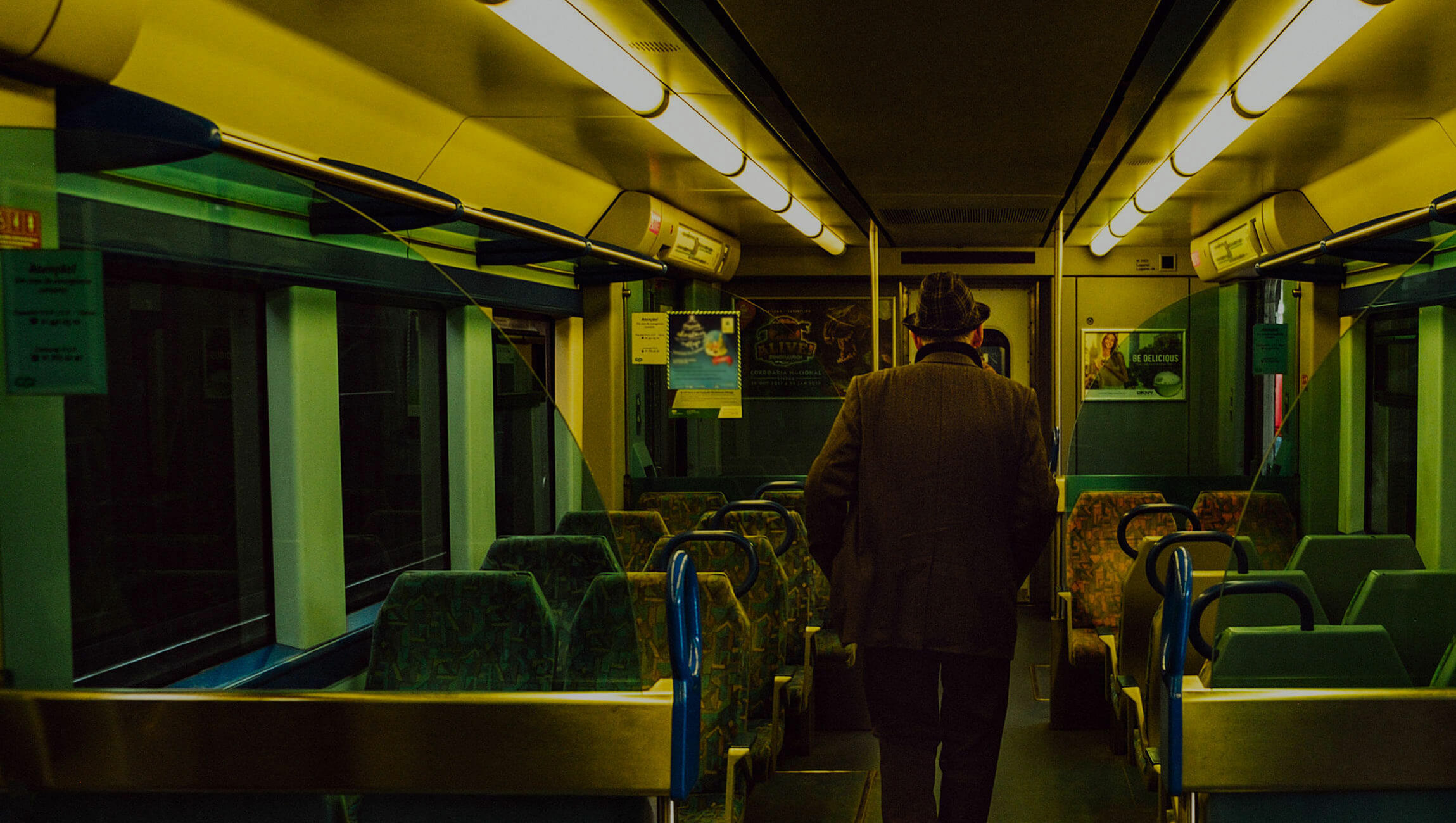
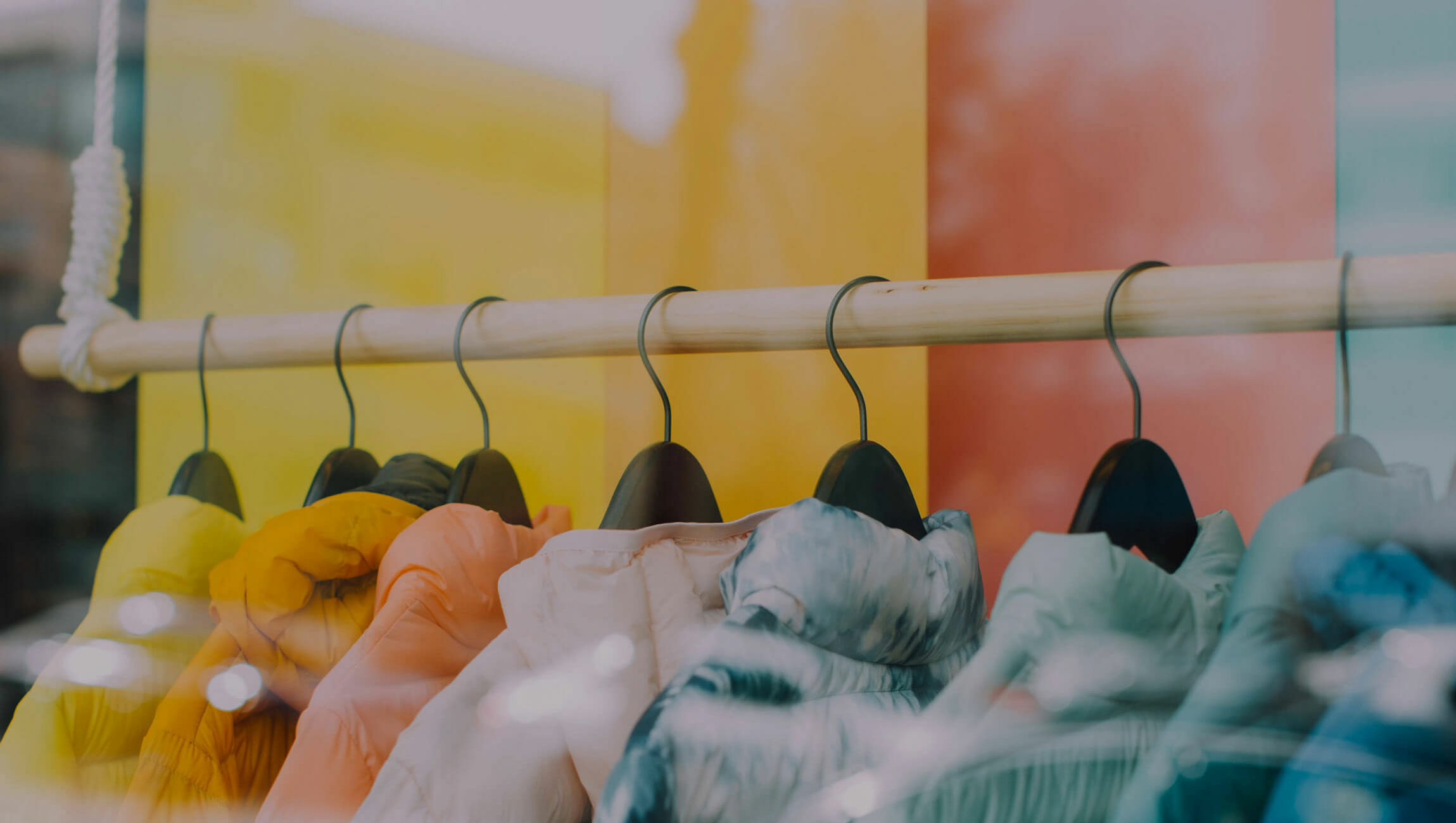
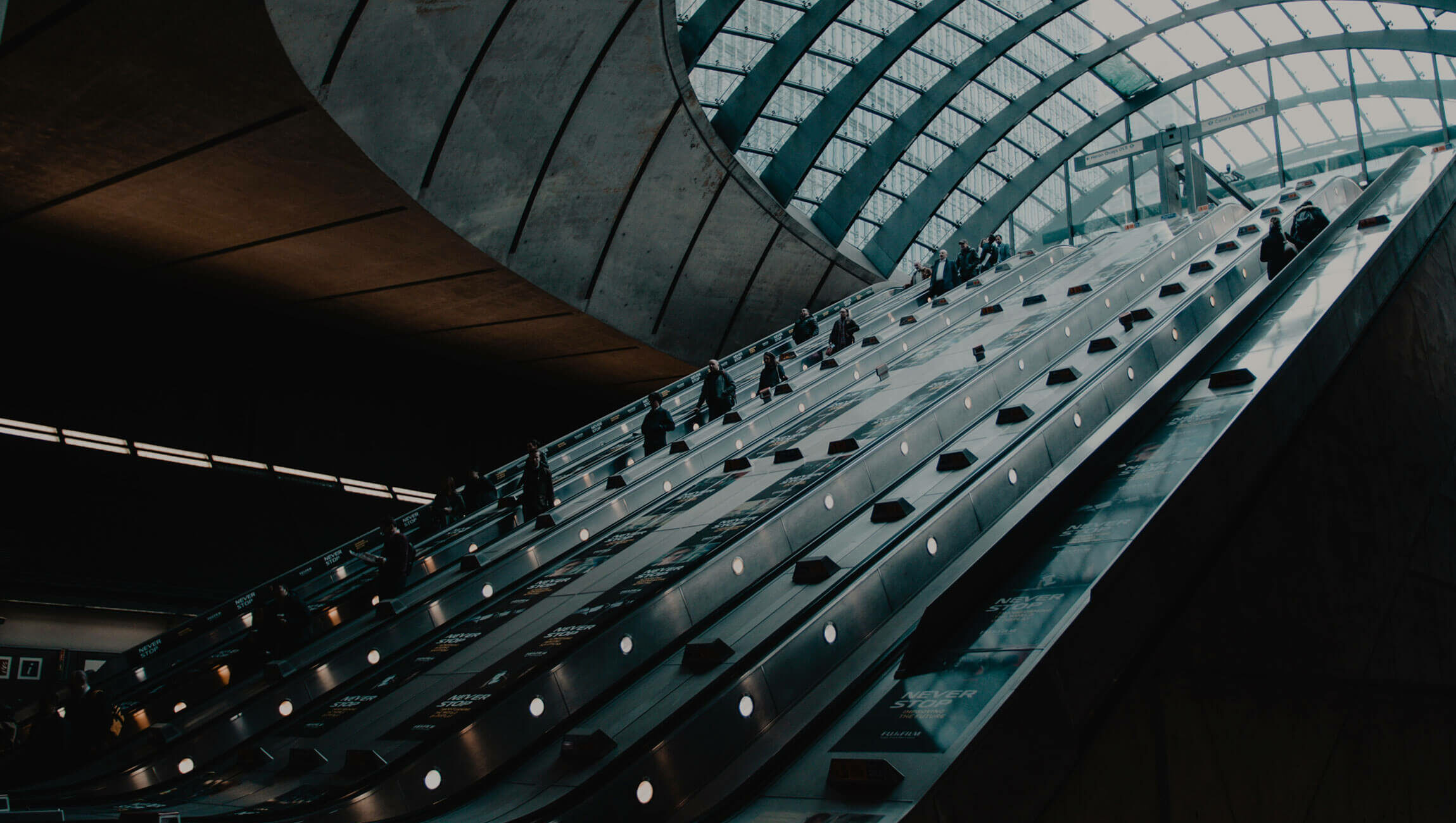
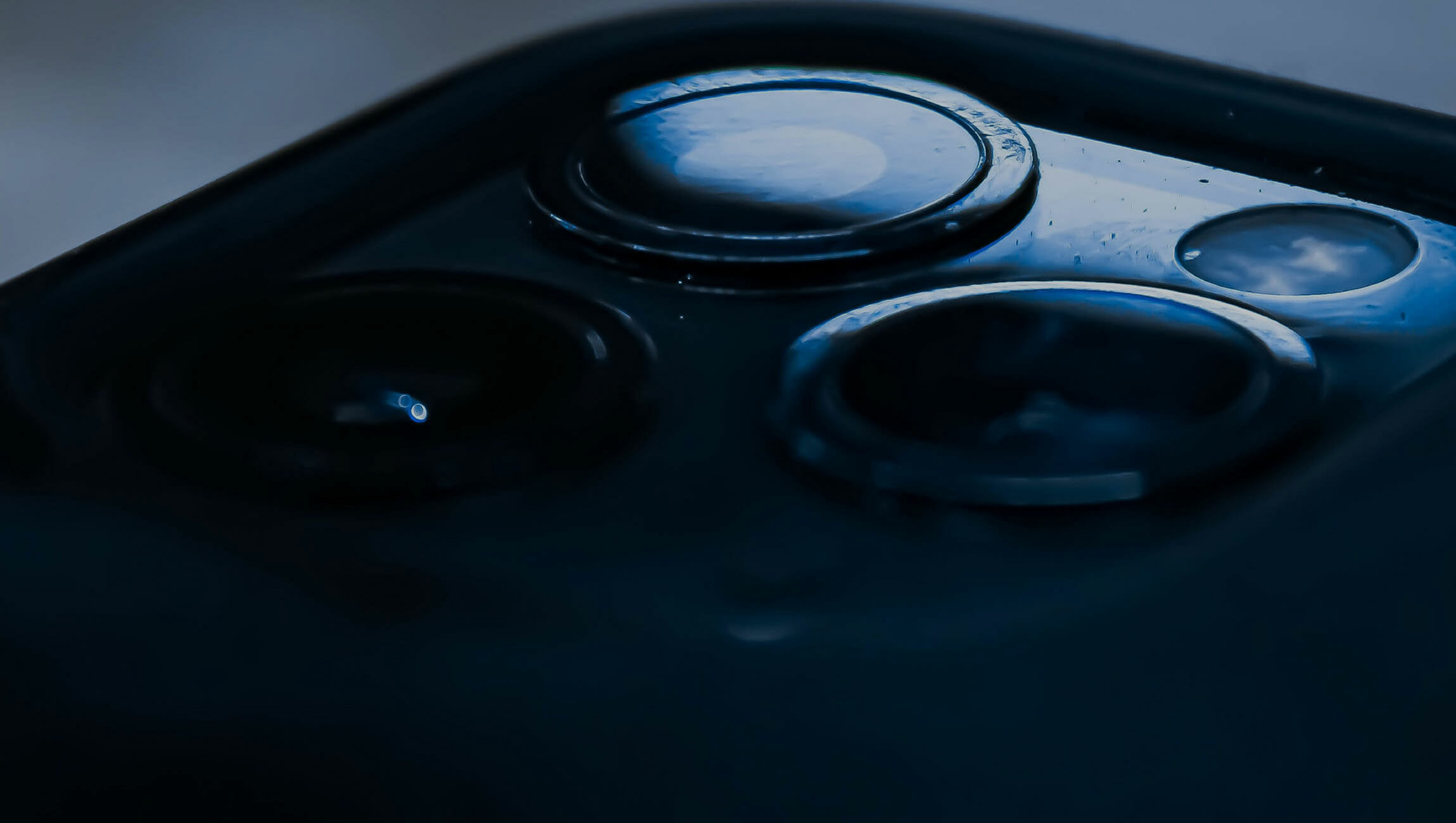
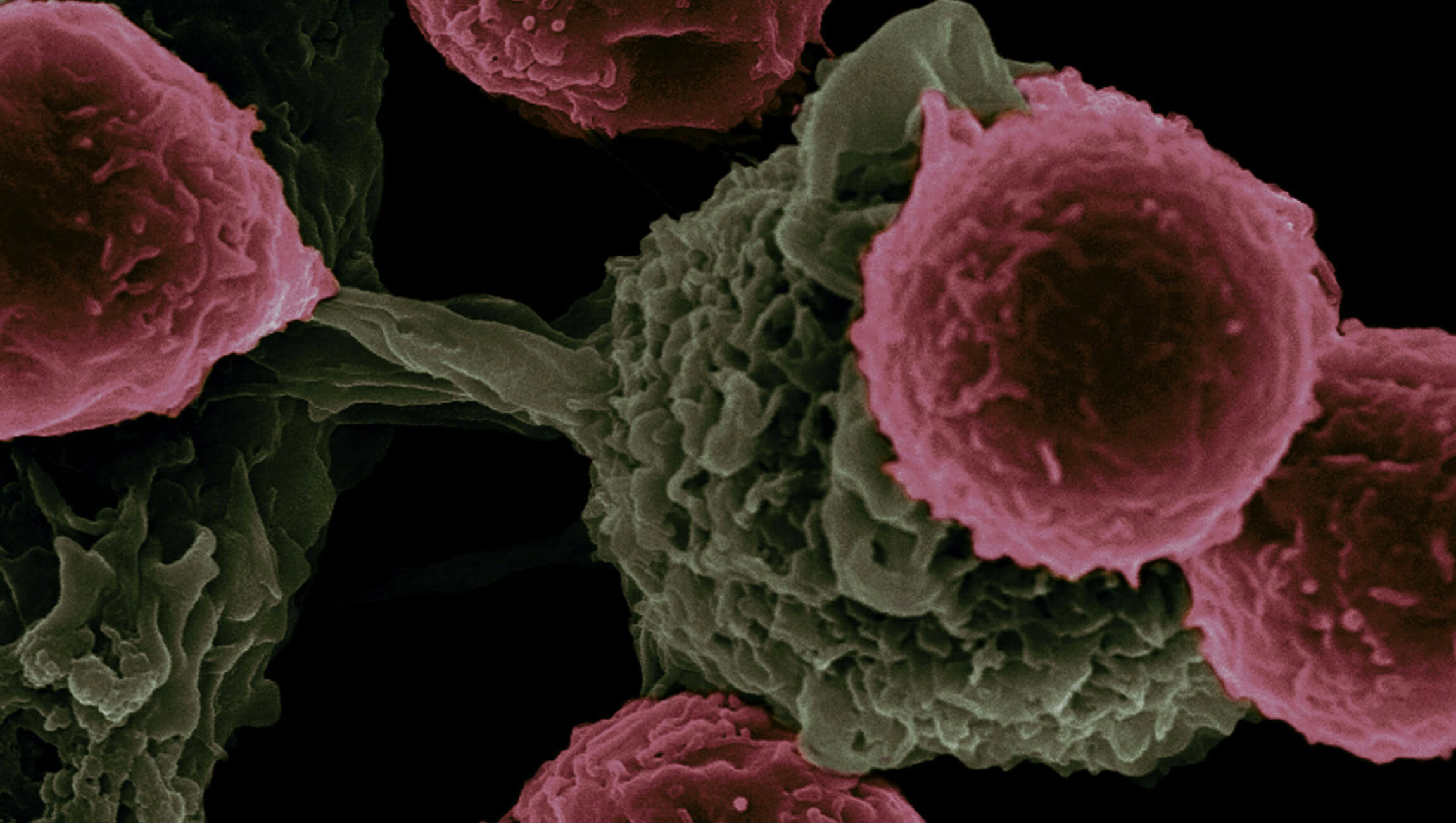
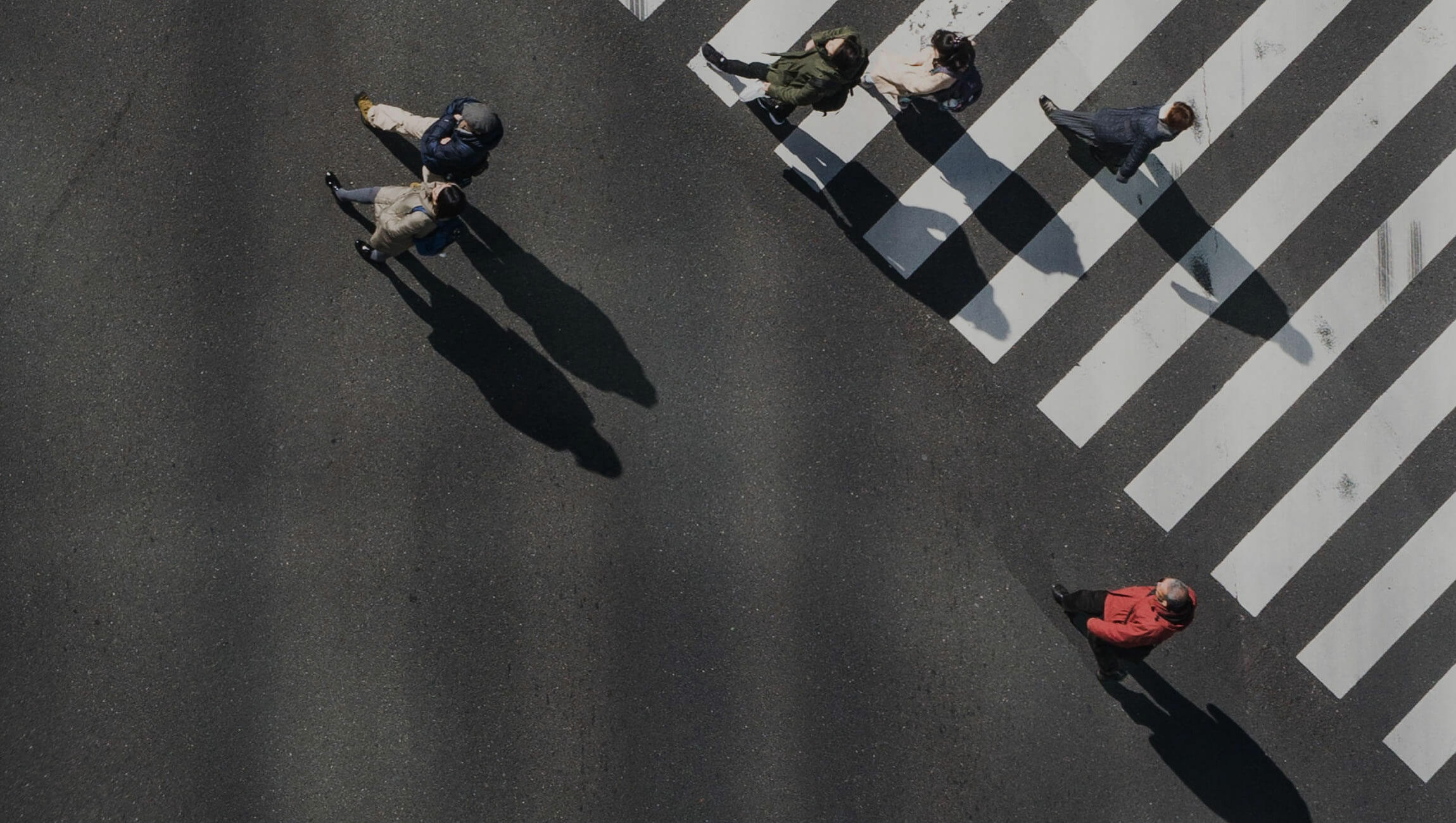
Impossible no more
We fuse AI into the DNA of your organization, creating and scaling self-learning systems that reinvent the very core of how you think, operate and innovate.
By combining the strengths of Human and Artificial Intelligence, we enable your teams to continually advance performance to new, previously impossible horizons.
Learn moreData & AI transformation
Create exponential value through new, AI-powered revenue streams and business models.
Data & AI Strategy
Our artificial intelligence strategies aren't about keeping up with your competition. We operationalize data and AI opportunities to make you the pacesetter.
More about Data & AI StrategyCustomized AI
By creating scalable, self-learning systems with humans in the loop, we continuously advance the performance frontier of your AI use cases.
More about Customized AI SolutionsData Foundations
We break down data silos and provide you with actionable data that will accelerate and futureproof your AI-driven digital transformations.
More about Data FoundationsML Ops
By deploying and managing machine learning models at scale, we help you rapidly advance your AI vision from research to reality.
More about ML OpsData & AI Training
We work with you to build the knowledge and skills you need to develop the best AI use cases, so your data and AI investment starts delivering impact, faster.
More about Data & AI TrainingGenerative AI (GenAI)
By deeply embedding GenAI into your culture and processes, we maximize its potential to unlock breakthrough-level benefits for your business.
More about Gen AIData & AI Strategy
Our artificial intelligence strategies aren't about keeping up with your competition. We operationalize data and AI opportunities to make you the pacesetter.
More about Data & AI StrategyCustomized AI
By creating scalable, self-learning systems with humans in the loop, we continuously advance the performance frontier of your AI use cases.
More about Customized AI SolutionsData Foundations
We break down data silos and provide you with actionable data that will accelerate and futureproof your AI-driven digital transformations.
More about Data FoundationsML Ops
By deploying and managing machine learning models at scale, we help you rapidly advance your AI vision from research to reality.
More about ML OpsData & AI Training
We work with you to build the knowledge and skills you need to develop the best AI use cases, so your data and AI investment starts delivering impact, faster.
More about Data & AI TrainingGenerative AI (GenAI)
By deeply embedding GenAI into your culture and processes, we maximize its potential to unlock breakthrough-level benefits for your business.
More about Gen AIB2B Sales Optimization Engine
What if we could use AI to deliver 100X lifetime ROI?
Supply Chain
What if we could use AI to reduce working capital by 18%?
Pricing & Revenue Management Solutions
What if we could use AI to increase annual contract value by 10%?
Promotion Optimization Engine
What if we could use AI to enhance bottom-line value by 30%?
We use AI to answer
Why our purpose matters.
‘what if?’ with ‘we can’.
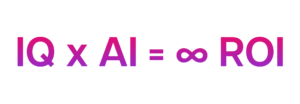
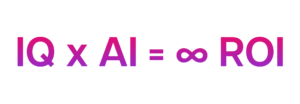
AI scalability requires AI capability.
Discover the programs we have built to fuse AI into your DNA at every level of your organisation.
We are Data & AI partner to the leaders of tomorrow.
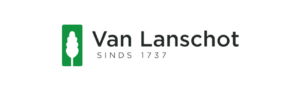
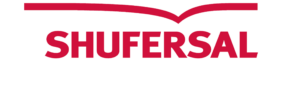
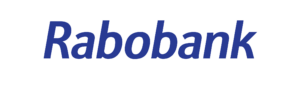
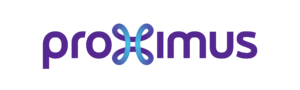
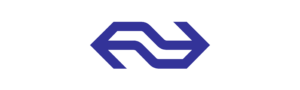
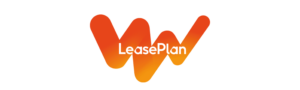
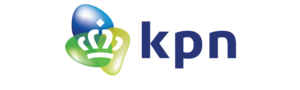
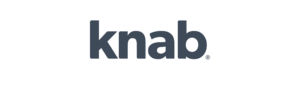
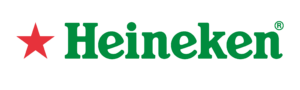
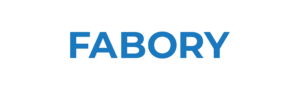
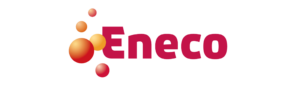
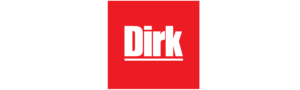
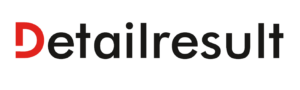
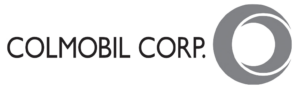
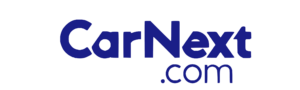
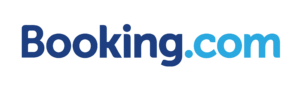
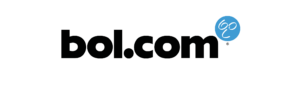
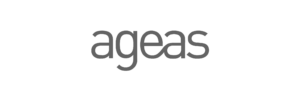
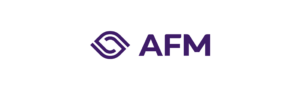
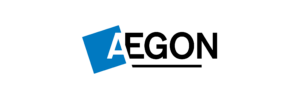
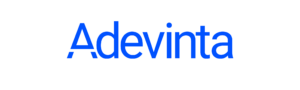
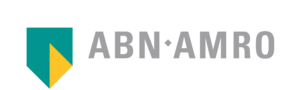
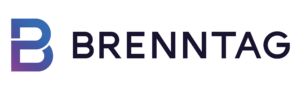
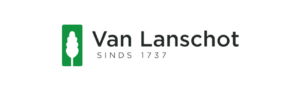
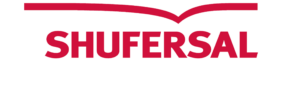
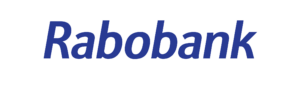
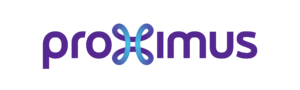
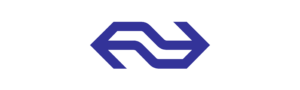
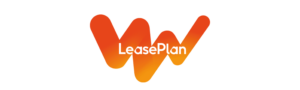
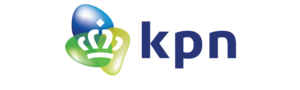
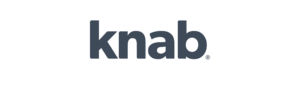
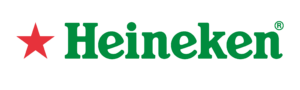
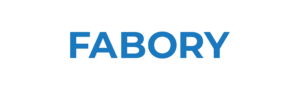
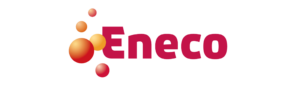
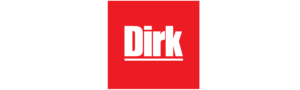
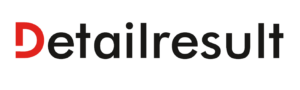
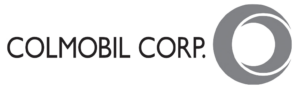
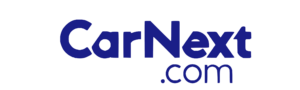
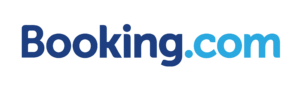
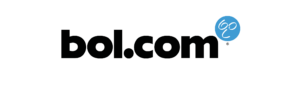
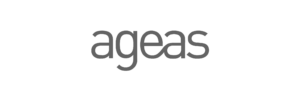
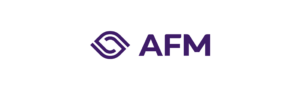
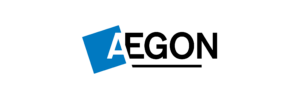
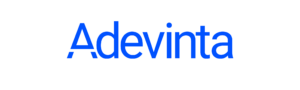
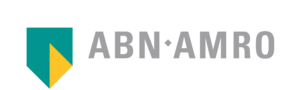
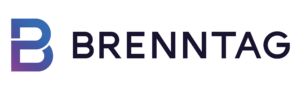